Machine Learning in F1: Driver Data Insights
Explore how machine learning revolutionizes Formula 1, enhancing race strategies, driver performance analysis, and real-time decision-making.
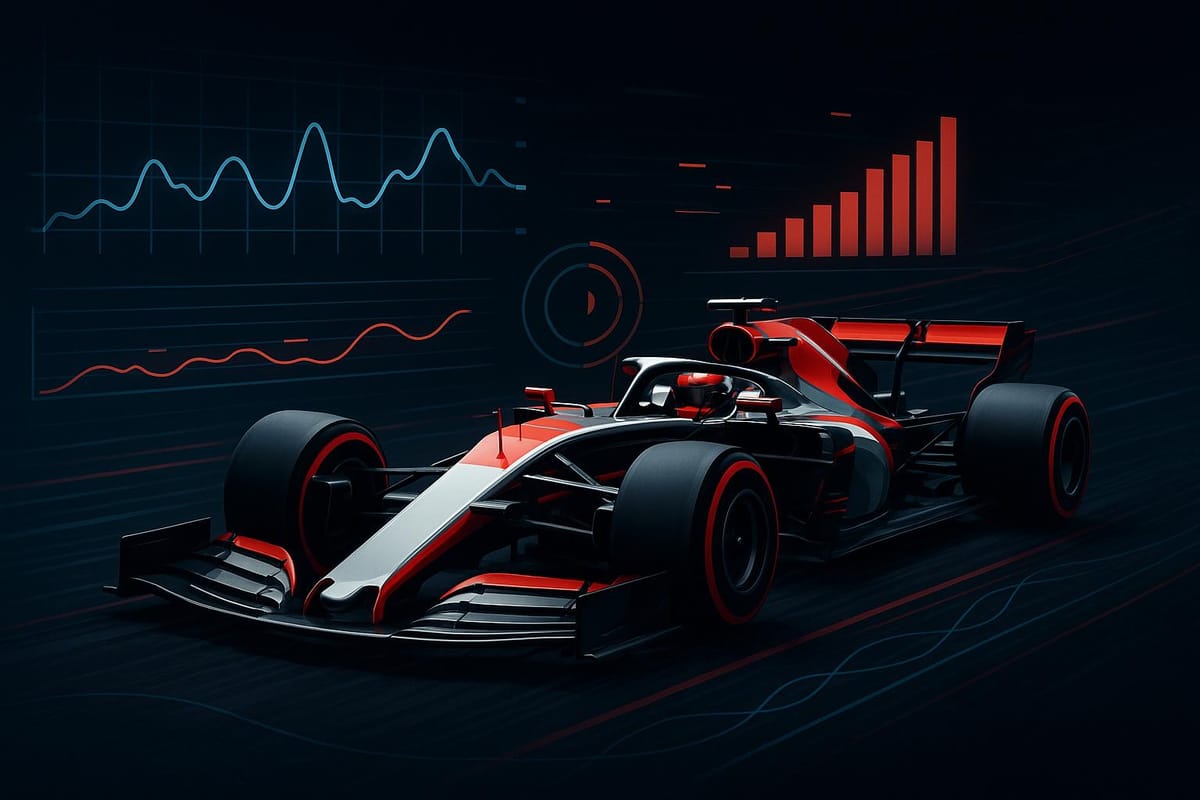
Machine learning (ML) is transforming Formula 1 by turning millions of data points into race-winning strategies. Here's how:
- Massive Data: F1 cars generate 1.1 million telemetry points every second from 300+ sensors. Teams analyze up to 160TB of data during a race weekend.
- Real-Time Insights: ML helps teams optimize braking, acceleration, and cornering while predicting race scenarios like pit stops or safety cars.
- Driver Performance Analysis: Sensors track driver biometrics and car performance, providing teams with actionable insights during races.
- Historical Comparisons: AI ranks drivers across eras, considering factors like lap times and teammate comparisons.
- Faster Decisions: Tools like AWS SageMaker and Oracle Cloud allow teams to process simulations and data analysis in hours instead of days.
Quick Facts:
- Mercedes processes 11TB of data per race weekend.
- Red Bull uses ML for 1.82-second pit stops.
- ML tools predict tire wear, fuel consumption, and mechanical failures.
Machine learning is reshaping F1, but human intuition and expertise remain key to success.
Data Systems and ML Setup
Car Sensors and Data Systems
Modern Formula 1 cars are technological marvels, equipped with about 300 sensors linked by nearly a mile of wiring. These sensors gather an astonishing 1.1 million telemetry points per second to monitor performance metrics in real time. Here's a quick breakdown of the data system's capabilities:
- Monitoring 10,000 individual data points per second
- Connecting to over 500 IoT devices
- Generating 30 megabytes of live data per lap
The collected data is encrypted and sent via radio frequency from a car-mounted antenna directly to the pit wall. This secure, continuous transmission ensures teams can analyze performance data instantly, which is crucial for making split-second decisions during a race.
Data Management Systems
Handling this massive volume of data requires sophisticated systems designed for real-time processing and analysis. To give you an idea of the scale, Mercedes generated an incredible 11 terabytes of data during the 2022 Mexico Grand Prix weekend alone.
"Connectivity is probably the lifeblood of the sport. Without it, nothing starts. No car can be on the track safely."
- Dan Keyworth, McLaren's director of business technology
Each team employs tailored systems to manage and analyze their data effectively:
- Mercedes-AMG Petronas relies on HPE's Apollo compute system, capable of processing up to 50 TB of CFD (Computational Fluid Dynamics) data weekly.
- Red Bull Racing uses Oracle's cloud platform to run detailed race simulations.
- Aston Martin Aramco utilizes data lakes to identify patterns and make predictions.
Current ML Models
Once the data is collected and organized, machine learning (ML) models come into play, transforming raw information into actionable insights. Tools like AWS SageMaker help teams build, train, and deploy these models efficiently.
"By adopting this tech, we are going to be able to free up multiple engineers so they can focus more acutely on car performance."
- Clare Lansley, Aston Martin Aramco's chief information officer
Some key ML applications in Formula 1 include:
- RaceWatch: Mercedes uses this tool for in-depth pre- and post-race analysis.
- AWS Integration: Formula 1 combines live telemetry with historical race data using Amazon S3, enabling real-time insights.
- Predictive Analytics: Teams leverage ML algorithms to anticipate race scenarios and optimize pit stop timing.
These advanced systems and tools underscore how Formula 1 teams are blending cutting-edge technology with high-speed competition to gain a crucial edge on race day.
ML Uses in Driver Analysis
Race Strategy Predictions
Machine learning (ML) has completely changed how Formula 1 teams predict and fine-tune race strategies. By analyzing a mix of data - like tire wear, fuel consumption, and competitors' positions - ML tools provide instant tactical insights. One key application is determining the perfect timing for pit stops, a decision that can make or break a race.
Take the 2019 British Grand Prix, for example. Mercedes relied on data-driven insights during a safety car period to make a crucial pit stop decision. This strategy played a pivotal role in securing Lewis Hamilton's victory.
"F1 can now deliver more data-driven insights that help to educate and entertain fans - from timing and telemetry data captured by hundreds of sensors on each F1 car, relayed real time to AWS compared to historical data stored on Amazon Simple Storage Service (Amazon S3). This includes comprehensive performance analysis and predictions used to drive the F1 Insights broadcast graphics." - Neil Ralph, AWS principal sports partnership manager
These predictive tools also extend seamlessly into real-time performance monitoring, offering teams a constant stream of actionable data.
Live Driver Performance Data
Every Formula 1 car is a rolling data hub, transmitting real-time performance data throughout the race. Teams monitor:
- Driver biometrics: Racing gloves equipped with sensors track vital signs.
- Car performance metrics: Constant updates on vehicle health and efficiency.
- Race conditions: Information used to adjust strategies on the fly.
ML models process this flood of information to anticipate potential issues, such as mechanical failures, allowing teams to adapt proactively. Over an entire race weekend, teams analyze millions of data points to fine-tune strategies and provide drivers with the best support possible.
But the impact of ML isn't just limited to live races - it also plays a key role in analyzing historical performance.
Cross-Era Driver Analysis
AWS's ML technology takes a deep dive into driver performance across different eras of Formula 1. Using the Massey method, it evaluates metrics like average lap time differences, teammate comparisons, and qualifying results. The system also filters out anomalies like accidents, mechanical breakdowns, and unpredictable weather.
This approach has led to rankings of the sport's fastest drivers, including:
- Ayrton Senna
- Michael Schumacher
- Lewis Hamilton
- Max Verstappen
- Fernando Alonso
"With machine learning, there are a number of opportunities to apply the technology to answer complex problems, and in this case, we hope to help settle age-old disputes with fans by using data to inform decisions." - Dr. Priya Ponnapalli, Principal Scientist and Senior Manager, Amazon ML Solutions Lab
Interestingly, the same advanced modeling techniques used for these rankings also help teams evaluate and select new drivers.
The Amazing Role Of Data, AI And Cloud In Formula 1
Risks and Ethics
Formula 1's growing use of machine learning (ML) for driver analysis brings with it a mix of opportunities and challenges. While these systems can boost performance, they also raise ethical and operational concerns that teams must address to maintain a fair and competitive environment.
Data Protection
The data used in F1, such as biometric and strategic information, is highly sensitive. Teams need to establish strong safeguards to protect this data and ensure compliance with relevant regulations. Beyond just securing the data, addressing potential biases in ML models is equally important to ensure fair outcomes.
ML Model Bias
One of the biggest risks with ML in F1 is the potential for societal biases to influence driver evaluations. Michael Sandel highlights this issue:
"Part of the appeal of algorithmic decision-making is that it seems to offer an objective way of overcoming human subjectivity, bias, and prejudice. But we are discovering that many of the algorithms that decide who should get parole, for example, or who should be presented with employment opportunities or housing … replicate and embed the biases that already exist in our society."
In F1, these biases can take several forms:
Bias Type | Impact on F1 | Mitigation Strategy |
---|---|---|
Data Bias | Skewed insights due to over- or underrepresentation of specific racing conditions | Collect diverse and representative data |
Algorithmic Bias | Distorted performance predictions based on historical patterns | Perform regular audits and apply fairness constraints |
Temporal Bias | Inaccurate comparisons across different racing eras | Use contextual analysis to account for changes in technology and regulations |
For example, when comparing drivers from different time periods, algorithms must consider advancements in car technology and shifts in race regulations that could affect performance metrics. Without this context, evaluations risk being unfair or misleading.
The issue of bias becomes even more pressing as AI investments grow. By 2025, global business spending on AI is expected to hit $50 billion. To address these challenges, F1 teams are focusing on:
- Frequent audits to ensure models are fair and accurate
- Openly reporting the limitations and assumptions behind their algorithms
- Collecting and analyzing data that reflects a wide range of scenarios
- Establishing clear ethical guidelines for AI use in motorsport
Many teams are now adopting bias-aware algorithms, embedding fairness constraints into the training process. These systems are routinely monitored and adjusted to tackle any new biases that may arise. Such efforts are critical to maintaining the fairness and accuracy that F1 demands.
Conclusion: Next Steps for F1 ML
Machine learning is reaching a pivotal moment in Formula 1, as teams increasingly rely on AI to redefine how they analyze driver performance and craft race strategies. Building on the detailed applications discussed earlier, recent examples highlight how these advancements are reshaping the sport.
In October 2024, GM Motorsports introduced AI-powered race strategy models capable of analyzing vehicle performance, track conditions, and competitor behavior in real time. Jonathan Bolenbaugh, Manager of Data and Analytics for GM Motorsports, shared his perspective:
"We're doing these things to cover the basics so the race engineers can do the things that only the humans can do... that intuition and that subject matter expertise still very much matters".
The FIA is also embracing AI to enhance race control and stewarding across F1, F2, and F3 series. By incorporating AI tools, the organization aims to ensure more consistent and fair race management. Francois Sicard, FIA single-seater operations director, elaborates:
"It can help race control for example to spot any track limits situation. Artificial Intelligence can help you detect [transgressions]. At the moment it's done by human beings, but in the future, you can imagine that it's done by Artificial Intelligence".
Teams are increasingly automating routine tasks with machine learning, enabling engineers to focus on critical performance areas. Looking ahead, these advancements are set to evolve further to improve race strategies, driver performance, and safety measures:
Area | Current Focus | Future Developments |
---|---|---|
Race Strategy | Real-time data analysis | Predictive modeling with greater precision |
Driver Performance | Sensor-based feedback | AI-driven personalized coaching systems |
Safety Systems | Component failure prediction | Proactive risk management and prevention |
With the $135 million USD cost cap for 2025, teams must strategically integrate machine learning to stay competitive without exceeding budget limits.
While machine learning offers advanced tools and insights, it complements - rather than replaces - human expertise. The intuition, experience, and decision-making of engineers and drivers remain irreplaceable, with AI serving to amplify their capabilities. This blend of human ingenuity and technological progress sets the stage for the next chapter in Formula 1's evolution.
FAQs
How does machine learning help Formula 1 teams make better race strategy decisions?
How Machine Learning Shapes Formula 1 Strategies
In Formula 1, machine learning has become a game-changer, enabling teams to fine-tune their race strategies by processing enormous amounts of data in real-time. Using advanced algorithms, teams can predict crucial elements like tire degradation, fuel consumption, and the optimal moments for pit stops. This allows them to adjust their tactics dynamically as the race unfolds.
Machine learning doesn’t stop there. Teams run thousands of simulations to explore various race scenarios, from identifying the best opportunities for overtaking to planning defensive maneuvers. These insights help them make split-second decisions with unmatched accuracy. By combining data-driven analysis with on-the-spot strategy, machine learning has added an entirely new level of precision and complexity to the high-stakes world of Formula 1.
How do Formula 1 teams ensure fairness in their machine learning models?
Formula 1 teams are actively working to reduce bias in their machine learning models to ensure their insights are both accurate and fair. A major part of this effort involves applying fairness metrics during model development. These metrics help pinpoint and address biases in the training data, ensuring the models are built on a balanced foundation. Another common approach is rebalancing datasets to include a wider range of scenarios, which enhances the model's ability to perform reliably under different conditions.
On top of that, many teams are turning to causal modeling to untangle the intricate relationships within their data. By focusing on this method, they can design datasets that preserve key features while minimizing bias. This not only makes AI decisions more transparent but also builds trust in the outcomes. These strategies help ensure that machine learning models capture the complexities of real-world racing while maintaining fairness in their evaluations.
How is machine learning used to analyze and compare driver performance across different eras in Formula 1?
Machine learning has become a game-changer in comparing driver performance across different eras in Formula 1. By processing massive amounts of historical telemetry data, it digs into details like braking patterns, acceleration habits, cornering techniques, and lap times. This helps uncover trends and shifts in driving styles and strategies over the years.
Beyond just analysis, these algorithms can predict crucial race factors, such as tire wear, fuel efficiency, and the best timing for pit stops. This not only sharpens current performance evaluations but also gives fans and teams a deeper appreciation of how drivers from various generations measure up against one another.